
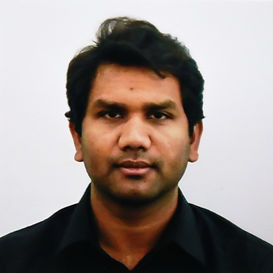
PRADEEP RAVINDRANATH
Computational chemist and Bioinformatician with over ten years of knowledge and experience in method development, software development, testing, validation and analysis. Broad range of knowledge in biology, physical chemistry, statistical mechanics and informatics. Strong written and oral communication skills. Expertise in applying computational techniques and tools to solve biological problems. Proven knowledge in interdisciplinary areas of research.
MY LATEST RESEARCH
AutoDock for Flexible Receptors (ADFR), a new protein-ligand docking engine based on the AutoDock4 scoring function that efficiently handles receptor flexibility. We have demonstrated that our software handles receptor sidechain flexibility with up to 14 sidechains efficiently increasing the success rate of docking in 2 scenarios 1) considering ligand diversity where 52 CDK2 ligands were docked into 1 apo structure requiring large conformational change in the binding site (4,10 and 12 flexible receptor sidechains) 2) considering receptor diversity where 17 ligands were docked into their corresponding apo receptor requiring large conformational change (upto 14 flexible receptor sidechains). We have also demonstrated that down-weighting the receptor internal energy will increase the success of docking, and highlighed that optimizing the scoring function when considering receptor flexibility requires further research. We are working on including additional receptor flexibility like backbone motion, loop conformational changes during protein-ligand docking.
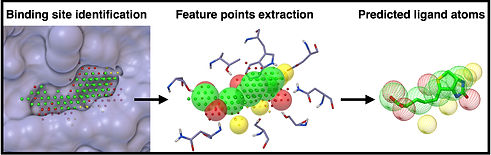
AutoSite is an efficient software tool for identifying ligand binding-sites and predicting pseudo ligand corresponding to each binding site identified. Binding-sites are reported as clusters of 3D points called fills in which every point is labeled as hydrophobic or as hydrogen bond donor or acceptor. From these fills AutoSite derives feature points: a set of putative positions of hydrophobic-, and hydrogen-bond-forming ligand atoms. We have demonstrated that AutoSite identifies ligand-binding sites with higher accuracy than other leading methods, and produces fills that better matches the ligand shape and properties, than the fills obtained with a software program with similar capabilities, AutoLigand. In addition, we have demonstrated that in Astex Diverse Set, the feature points identify 79% of hydrophobic ligand atoms, and 81% and 62% of the hydrogen acceptor and donor hydrogen ligand atoms interacting with the receptor, and predict 81.2% of water molecules mediating interactions between ligand and receptor. Finally we have illustrated the potential uses of the predicted feature points in the context of lead optimization in drug discovery projects.